Unlocking New Frontiers of Innovation
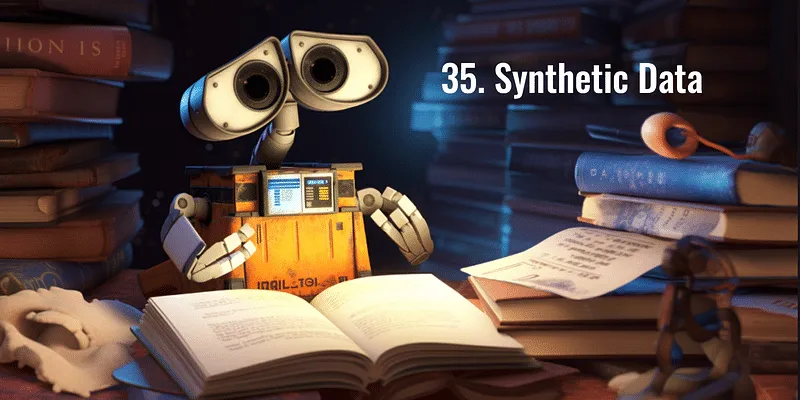
In the era of artificial intelligence (AI) and machine learning, data has emerged as the lifeblood of innovation. The ability to gather and analyze large volumes of data has opened new doors for businesses, researchers, and developers to create intelligent systems that can make accurate predictions and informed decisions. However, accessing high-quality and diverse datasets can be a challenging and resource-intensive task. This is where synthetic data comes into play. Synthetic data, generated by computer algorithms, has proven to be a valuable asset in overcoming the limitations of traditional data collection methods. In this article, we explore the concept of synthetic data and its potential in driving AI innovation.
Understanding Synthetic Data
Synthetic data refers to artificially generated data that simulates the characteristics and statistical properties of real-world data. It is created using algorithms or models that mimic the patterns, relationships, and distributions found in actual datasets. Synthetic data can be used as a substitute for or in combination with real data, offering several advantages in terms of privacy, scalability, and diversity.
Benefits of Synthetic Data
Privacy Preservation: Privacy concerns have become increasingly important as more data is collected and shared. Synthetic data provides a solution by generating data that is entirely fictional but still retains the statistical properties of the original dataset. This allows researchers and organizations to work with sensitive data without compromising individual privacy.
Scalability and Accessibility: Collecting and curating large-scale datasets can be time-consuming, costly, and limited by factors such as legal restrictions or geographical boundaries. Synthetic data overcomes these limitations by providing an unlimited supply of generated data that can be tailored to specific requirements. This enables researchers and developers to experiment with AI models on a larger scale, fostering innovation and accelerating progress.
Data Diversity and Representation: Real-world datasets often suffer from biases, resulting in inadequate representation of certain groups or demographics. Synthetic data can be designed to address these biases, ensuring a more diverse and representative dataset for training AI models. By capturing a broader range of scenarios and examples, synthetic data helps mitigate bias and enhances the robustness and fairness of AI systems.
Experimental Flexibility: Synthetic data allows researchers and developers to create controlled environments for testing AI algorithms and models. By generating datasets with specific characteristics, researchers can explore various scenarios, anomalies, or edge cases that may be difficult or time-consuming to encounter in real-world data. This flexibility enables faster iterations and improvements in AI systems.
Applications of Synthetic Data
Healthcare and Medical Research: Synthetic data has the potential to revolutionize healthcare by generating large-scale, privacy-preserving datasets for medical research. It can help facilitate AI-driven diagnostics, drug development, and patient monitoring, while ensuring the confidentiality of sensitive patient information.
Autonomous Vehicles: Training self-driving cars requires vast amounts of diverse and realistic data. Synthetic data can augment real-world driving data by generating virtual scenarios and traffic situations, allowing AI systems to learn and adapt in a safe and controlled environment.
Cybersecurity: Synthetic data can aid in detecting and preventing cyber threats by generating realistic datasets that simulate attack patterns and network behavior. This enables the development and testing of robust AI-powered cybersecurity solutions.
Financial Services: Synthetic data can be used in the financial industry to enhance risk analysis, fraud detection, and algorithmic trading. By generating synthetic financial transactions, researchers and organizations can create realistic testbeds to validate the effectiveness of their algorithms.
Challenges and Future Directions
Despite its promising potential, synthetic data generation still faces challenges. Ensuring the generated data accurately reflects the complexities of the real world remains a significant hurdle. Creating models that capture nuanced behaviors, interactions, and contexts is an ongoing research endeavor.
Additionally, the ethical implications of using synthetic data should be carefully considered, including the potential for unintended biases or the impact on decision-making systems trained solely on artificial data.
Synthetic data has emerged as a powerful tool in the realm of AI and machine learning. By addressing challenges related to privacy, scalability, diversity, and experimentation, synthetic data opens up new possibilities for innovation across various industries. As researchers and developers continue to refine synthetic data generation techniques and explore its applications, we can expect it to play a vital role in shaping the future of AI-driven technologies.