AI Terminology 101: One-Shot Learning
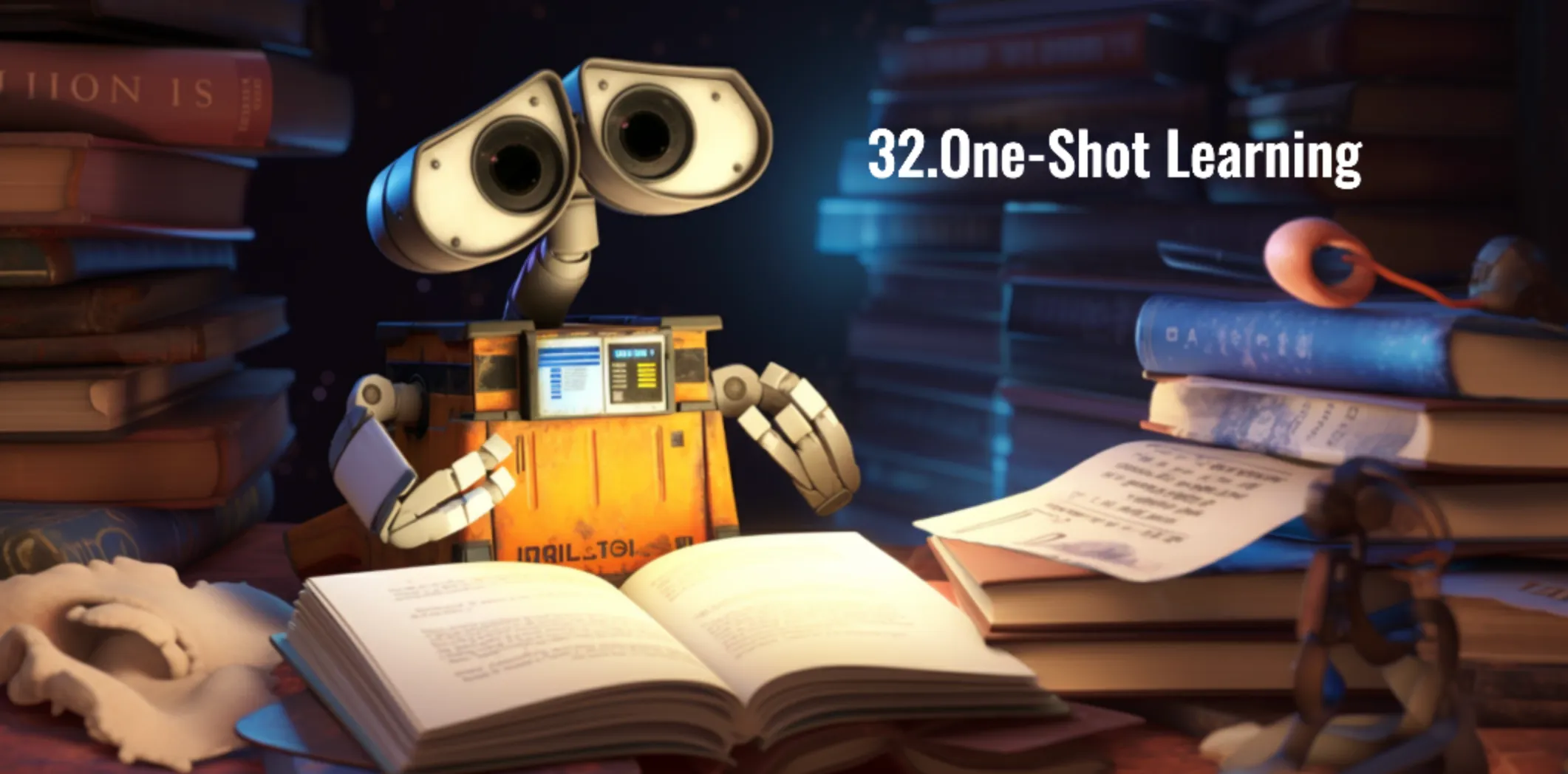
Artificial intelligence (AI) continually strives to mimic human-like learning capabilities, and one such exciting concept is One-Shot Learning. Unlike traditional machine learning methods that require a large amount of labeled data, One-Shot Learning enables machines to learn from just a single example. In this article, we delve into the world of One-Shot Learning and uncover its mechanics, applications, and impact it has on the field of AI.
Understanding One-Shot Learning
One-Shot Learning is an approach that allows machines to learn and generalise from a single or very few instances. Human beings possess the remarkable ability to recognise and understand new concepts with just a single exposure. One-Shot Learning aims to replicate this capability in machines by enabling them to identify and classify novel objects, individuals, or patterns with minimal training data.
Traditional machine learning models rely on a vast amount of labeled data to establish patterns and make accurate predictions. However, in real-world scenarios, acquiring extensive labeled datasets can be challenging, time-consuming, or even impossible. One-Shot Learning offers a solution to this limitation, as it equips machines with the ability to learn quickly and generalise from limited examples.
Mechanisms Behind One-Shot Learning
One-Shot Learning algorithms employ various techniques to enable machines to generalise from sparse data. These techniques include siamese networks, metric learning, and generative models. Siamese networks learn to measure the similarity between two inputs, allowing for effective comparisons and recognition of novel instances. Metric learning focuses on learning a distance metric that captures the similarity or dissimilarity between samples. Generative models generate new instances of a given class from a limited set of examples, facilitating better generalisation.
Applications of One-Shot Learning
One-Shot Learning has far-reaching implications across multiple domains. Computer vision enables machines to recognise and classify objects or people based on a single image or a few examples. This has applications in face recognition, object detection, and image classification. In natural language processing, One-Shot Learning can assist in language translation, sentiment analysis, and text generation tasks, even
with limited training data.
One-Shot Learning also finds utility in robotics, where robots can quickly adapt to new environments and objects with minimal prior knowledge. It enables personalised recommendation systems to make accurate suggestions based on limited user preferences. Furthermore, in healthcare, One-Shot Learning aids in the diagnosis of rare diseases by learning from a small number of patient cases.
The Future of One-Shot Learning
As the field of AI progresses, One-Shot Learning continues to be an active area of research and development. Researchers are exploring techniques to enhance the performance and robustness of One-Shot Learning models. Advancements in generative models, transfer learning, and meta-learning are expanding the possibilities of One-Shot Learning and its potential for real-world applications.
Embrace the Power of One-Shot Learning
One-Shot Learning revolutionises the field of AI by enabling machines to learn from limited examples, mirroring the human ability to generalise quickly. Its potential to address data scarcity and facilitate rapid learning from sparse data opens up new avenues for AI applications. Embracing One-Shot Learning empowers organisations to build more flexible and adaptable AI systems with minimal training data.
In our future articles, we’ll continue to explore other AI terminologies, including Reinforcement Learning, Deep Reinforcement Learning, and Explainable AI. Stay tuned as we unravel the mysteries of AI, unveiling its transformative potential in diverse domains.