AI Terminologies 101: Bayesian Networks
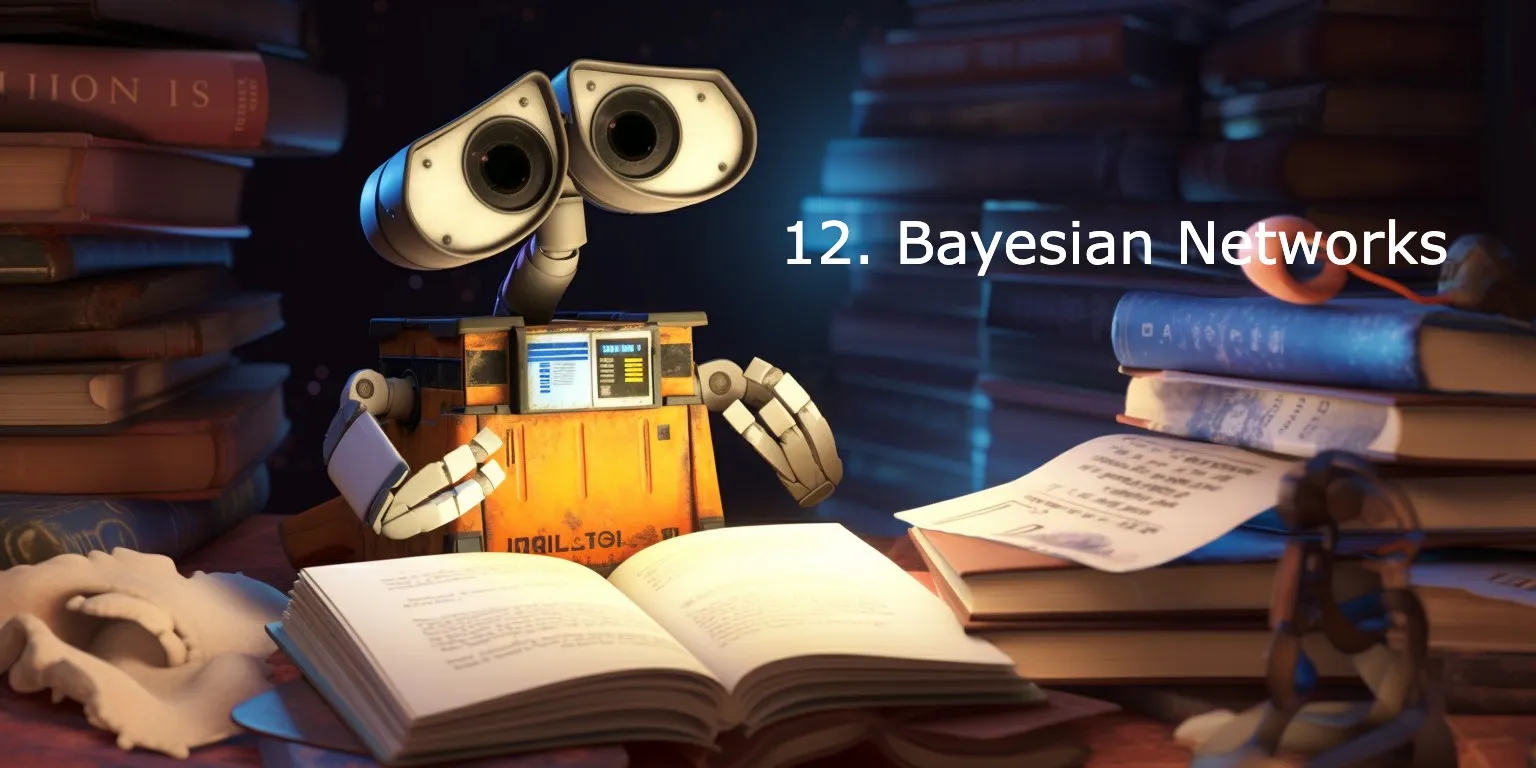
Bayesian Networks, also known as Belief Networks or Bayes Nets, are a powerful probabilistic graphical model used for reasoning under uncertainty. They have been successfully applied to a wide range of tasks, including diagnostics, decision support systems, and natural language processing. In this article, we will explore the concept of Bayesian Networks, their underlying principles, and their applications across various domains.
Body:
Bayesian Networks were introduced by Judea Pearl in the 1980s as a way to represent and reason with uncertain knowledge. They are based on the principles of Bayesian probability theory and provide a compact representation of complex probabilistic relationships among variables. A Bayesian Network is a directed acyclic graph (DAG) consisting of nodes, which represent random variables, and edges, which represent conditional dependencies between these variables.
The primary strength of Bayesian Networks lies in their ability to model uncertain relationships and make probabilistic inferences. They are particularly useful in situations where there is limited or noisy data, as they can still provide reliable estimates of the probabilities of different outcomes.
Bayesian Networks can be used for various tasks, including:
- Prediction: Estimating the probability of a variable’s value given the values of other variables.
- Diagnosis: Identifying the most likely cause of an observed outcome.
- Decision making: Evaluating the expected utility of different actions and choosing the optimal action.
Bayesian Networks have been employed in diverse applications across multiple domains. In medicine, they have been used for disease diagnosis and treatment planning, taking into account various symptoms, test results, and patient history. In natural language processing, Bayesian Networks can be used to model the relationships between words and extract meaning from text. In decision support systems, they can aid decision-makers by providing probabilistic estimates of the outcomes of different actions.
Conclusion:
Bayesian Networks offer a powerful and flexible approach to reasoning under uncertainty, with applications spanning various fields. Their ability to model complex probabilistic relationships and make reliable inferences, even with limited data, make them a valuable tool in the realm of artificial intelligence.
In future articles, we’ll dive deeper into other AI terminologies, like Swarm Intelligence, Evolutionary Algorithms, and Explainable AI. We’ll explain what they are, how they work, and why they’re important. By the end of this series, you’ll have a solid understanding of the key concepts and ideas behind AI, and you’ll be well-equipped to explore this exciting field further.
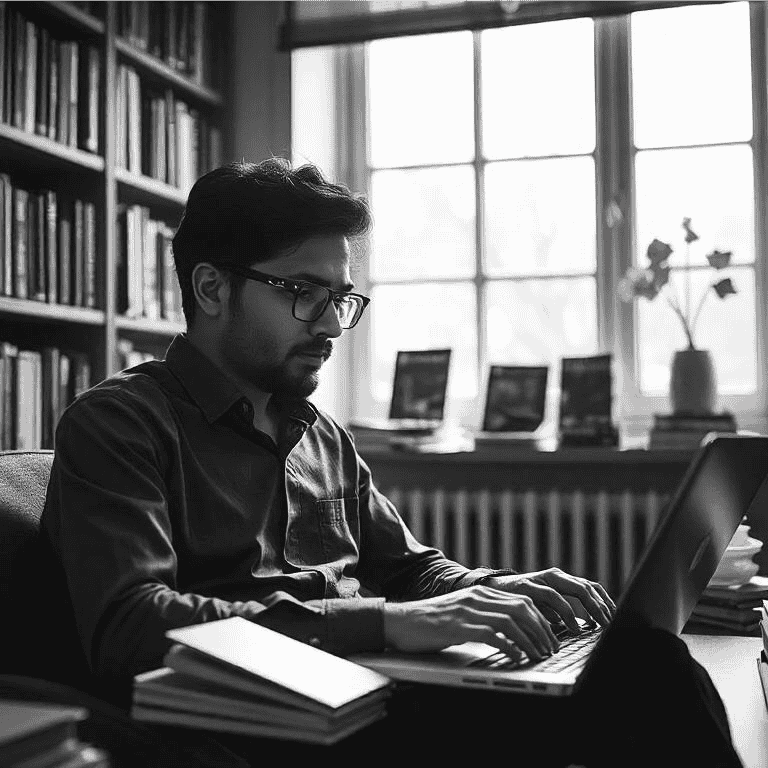
Atul Tiwari is a seasoned journalist at Mumbai Times, specializing in city news, culture, and human-interest stories. With a knack for uncovering compelling narratives, Atul brings Mumbai’s vibrant spirit to life through his writing.